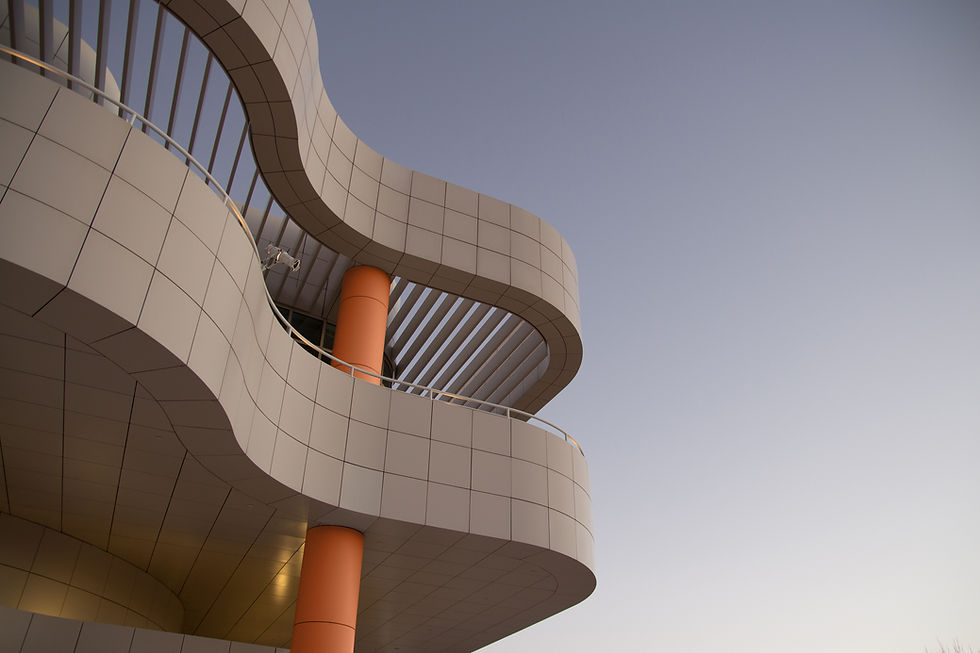
Research Overview: How To Perform Trusted Data Augmentation for Time Series Data?
Jul 25, 2024
2 min read
0
3
0
Balanced data is required for deep neural networks (DNNs) when learning to perform power system stability assessment. However, power system measurement data contains relatively few events from where power system dynamics can be learnt. To mitigate this imbalance, we propose a novel data augmentation strategy preserving the dynamic characteristics to be learnt. The augmentation is performed using Variational Mode Decomposition (VMD). The detrended and the augmented data are tested for distributions similarity using Kernel Maximum Mean Discrepancy test. In addition, the effectiveness of the augmentation methodology is validated via training an Encoder DNN utilizing original data, testing using the augmented data, and evaluating the Encoder’s performance employing several metrics.

Fig. 1. Examples of input signals
Hypothesis: Can the VMD-decomposed data (e.g. as illustrated in Fig. 1) be used as augmented data when the detrended data are used as input for a deep learning model, if the two distributions of the data are similar enough? In this case if the hypothesis is validated, the augmented data can be considered as trusted augmentation since the method of data processing is known and unambiguous, which helps with data credibility.
Conclusion:
By analogy to rotation, cropping, and flipping of an image, the decomposed components of the signal may serve as a means to augment the limited data set with valuable dynamics. To validate the hypothesis that VMD-decomposed data can serve as augmented data for the neural network, the KMMD statistical test of distribution similarity is performed. The results of the testing have shown that the VMD-decomposed data can be considered as augmented data under relaxed conditions posed by the Rademacher boundary. The additional validation of the proposed augmentation has been performed using TSTR and TRTS metrics. The outcome has shown good performance of the Encoder neural network used on the newly formed data. The Encoder has demonstrated a consistently positive performance with the increasing data size, which includes the additional “augmented” data.
For more details check: Bogodorova, Tetiana, Denis Osipov, and Luigi Vanfretti. "Variational Mode Decomposition as Trusted Data Augmentation in ML-based Power System Stability Assessment." arXiv preprint arXiv:2406.09235 (2024).